Welcome to the ICCV 2023 SLAM Challenge!
We provide datasets TartanAir and SubT-MRS, aiming to push the rubustness of SLAM algorithms in challenging environments, as well as advancing sim-to-real transfer. Our datasets contain a set of perceptually degraded environments such as darkness, airborne obscurant conditions such as fog, dust, smoke and lack of prominent perceptual features in self-similar areas. One of our hypotheses is that in such challenging cases, the robot needs to rely on multiple sensors to reliably track and localize itself. We provide a rich set of sensory modalities including RGB images, LiDAR points, IMU measurements, thermal images and so on.
Feel free to check our Paper and cite our work if it is useful ^ ^.
@InProceedings{Zhao_2024_CVPR,
author = {Zhao, Shibo and Gao, Yuanjun and Wu, Tianhao and Singh, Damanpreet and Jiang, Rushan and Sun, Haoxiang and Sarawata, Mansi and Qiu, Yuheng and Whittaker, Warren and Higgins, Ian and Du, Yi and Su, Shaoshu and Xu, Can and Keller, John and Karhade, Jay and Nogueira, Lucas and Saha, Sourojit and Zhang, Ji and Wang, Wenshan and Wang, Chen and Scherer, Sebastian},
title = {SubT-MRS Dataset: Pushing SLAM Towards All-weather Environments},
booktitle = {Proceedings of the IEEE/CVF Conference on Computer Vision and Pattern Recognition (CVPR)},
month = {June},
year = {2024},
pages = {22647-22657}
}
Time remaining:
SubT-MRS Dataset
The SubT-MRS Dataset is an exceptional real-world collection of challenging datasets obtained from Subterranean Environments, encompassing caves, urban areas, and tunnels. Its primary focus lies in testing robust SLAM capabilities and is designed as Multi-Robot Datasets, featuring UGV, UAV, and Spot robots, each demonstrating various motions. The datasets are distinguished as Multi-Spectral, integrating Visual, Lidar, Thermal, and inertial measurements, effectively enabling exploration under demanding conditions such as darkness, smoke, dust, and geometrically degraded environments.Key features of our dataset:
1. Multiple Modalities: Our dataset includes hardware time-synchronized data from 4 RGB cameras, 1 LiDAR, 1 IMU, and 1 thermal camera, providing diverse and precise sensor inputs.
2.Diverse Scenarios: Collected from multiple locations, the dataset exhibits varying environmental setups, encompassing indoors, outdoors, mixed indoor-outdoor, underground, off-road, and buildings, among others.
3.Multi-Degraded: By incorporating multiple sensor modalities and challenging conditions like fog, snow, smoke, and illumination changes, the dataset introduces various levels of sensor degradation.
4. Heterogeneous Kinematic Profiles: The SubT-MRS Dataset uniquely features time-synchronized sensor data from diverse vehicles, including RC cars, legged robots, drones, and handheld devices, each operating within distinct speed ranges.
Tartan Air Dataset
The TartanAir dataset is collected in photo-realistic simulation environments based on the AirSim project. A special goal of this dataset is to focus on the challenging environments with changing light conditions, adverse weather, and dynamic objects. The four most important features of our dataset are:
1. Large size diverse realistic data: We collect the data in diverse environments with different styles, covering indoor/outdoor, different weather, different seasons, urban/rural.
2. Multimodal ground truth labels: We provide RGB stereo, depth, optical flow, and semantic segmentation images, which facilitates the training and evaluation of various visual SLAM methods.
3. Diversity of motion patterns: Our dataset covers much more diverse motion combinations in 3D space, which is significantly more difficult than existing datasets.
4. Challenging Scenes: We include challenging scenes with difficult lighting conditions, day-night alternating, low illumination, weather effects (rain, snow, wind and fog) and seasonal changes.Please refer to the TartanAir Dataset and the paper for more information.
ICCV 2023 SLAM Challenge
🚀 The Challenge is Live! 🚀 Join us for three exciting tracks of challenges from the links below.
🚀 You can participate either Visual-inertial challenge or
LiDAR-inertial challenge Or participate in Sensor Fusion challenge.
Three separate awards will be given for each track. Your SLAM performance in the Sensor Fusion track will not impact the scores in other tracks.
Join us now to become a vital part of cutting-edge advancements in robotics and sensor fusion! 🤖💡 Let your expertise shine in this thrilling competition!
Meet our robots!!
Sensors
Sponsors
We thank Nvidia for supporting our challenge! The winning team will be awarded with an Orin Developer Kit and Orin Nano Developer Kit!
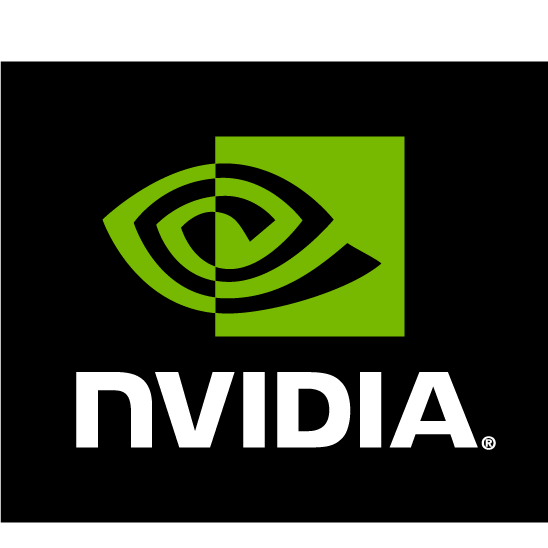
Contact us
If you have any question, please do not hesitate to post issues on this github website. We would love to hear from your feedback! Every post will be responded with no spared effort within 36 hours.
Relevant Work
Wenshan, Wang et al. “TartanAir: A Dataset to Push the Limits of Visual SLAM” Paper
@misc{wang2020tartanair,
title={TartanAir: A Dataset to Push the Limits of Visual SLAM},
author={Wenshan Wang and Delong Zhu and Xiangwei Wang and Yaoyu Hu and Yuheng Qiu and Chen Wang and Yafei Hu and Ashish Kapoor and Sebastian Scherer},
year={2020},
eprint={2003.14338},
archivePrefix={arXiv},
primaryClass={cs.RO}
}
Kamak Ebadi, et al. “Present and future of slam in extreme underground environments” Paper
@misc{ebadi2022present,
title={Present and Future of SLAM in Extreme Underground Environments},
author={Kamak Ebadi and Lukas Bernreiter and Harel Biggie and Gavin Catt and Yun Chang and Arghya Chatterjee and Christopher E. Denniston and Simon-Pierre Deschênes and Kyle Harlow and Shehryar Khattak and Lucas Nogueira and Matteo Palieri and Pavel Petráček and Matěj Petrlík and Andrzej Reinke and Vít Krátký and Shibo Zhao and Ali-akbar Agha-mohammadi and Kostas Alexis and Christoffer Heckman and Kasra Khosoussi and Navinda Kottege and Benjamin Morrell and Marco Hutter and Fred Pauling and François Pomerleau and Martin Saska and Sebastian Scherer and Roland Siegwart and Jason L. Williams and Luca Carlone},
year={2022},
eprint={2208.01787},
archivePrefix={arXiv},
primaryClass={cs.RO}
}
Organizers
![]() PhD Candidate Carnegie Mellon University |
![]() Master's Student Carnegie Mellon University |
![]() Research Associate Carnegie Mellon University |
![]() Undergradate Student Carnegie Mellon University |
![]() Undergradate Student Carnegie Mellon University |
![]() Senior Field Robotics Specialist Carnegie Mellon University |
![]() Graduate Student Carnegie Mellon University |
![]() Research Associate Carnegie Mellon University |
![]() Research Associate Carnegie Mellon University |
![]() Undergraduate Student Carnegie Mellon University |
![]() Research Associate Carnegie Mellon University |
![]() MSR Student Carnegie Mellon University |
![]() MSME Student Carnegie Mellon University |
![]() Research Associate Carnegie Mellon University |